The landscape of GenAI is rapidly evolving, and midmarket firms are striving to keep pace with this change. New data from Techaisle (SMB/Midmarket AI Adoption Trends Research) sheds light on a fascinating trend: adopting multiple large language models (LLMs), an average of 2.2, by core and upper midmarket firms. Data also shows that 36% of midmarket firms are piloting with an average of 3.5 LLMs, and another 24% will likely add another 2.2 LLMs within the year.
The survey reveals a preference for established players like OpenAI, with a projected penetration rate of 89% within the midmarket firms currently adopting GenAI. Google Gemini is close behind, with an expected adoption rate of 78%. However, the data also paints a picture of a dynamic market. Anthropic is experiencing explosive growth, with an anticipated adoption growth rate of 100% and 173% in the upper and core midmarket segments, respectively. A recent catalyst in midmarket interest for Anthropic is the availability of Anthropic’s Claude 3.5 Sonnet in Amazon Bedrock.
This trend towards multi-model adoption signifies a crucial step – midmarket firms are no longer looking for a one-size-fits-all LLM solution. They are actively exploring the functionalities offered by various models to optimize their specific needs.
However, the data also raises questions about the long-term sustainability of this model proliferation due to higher costs, demand for engineering resources (double-bubble shocks), integration challenges, and security. Additionally, market saturation might become a challenge with several players offering overlapping capabilities. Only time will tell which models will endure and which will fall by the wayside.
Furthermore, the survey highlights a rising interest in custom-built LLMs. An increasing portion of midmarket firms (11% in core and 25% in upper) will likely explore this avenue. In a corresponding study of partners, Techaisle data shows that 52% of partners offering GenAI solutions anticipate building custom LLMs, and 64% are building SLMs for their clients, indicating a potential shift towards smaller specialized solutions.
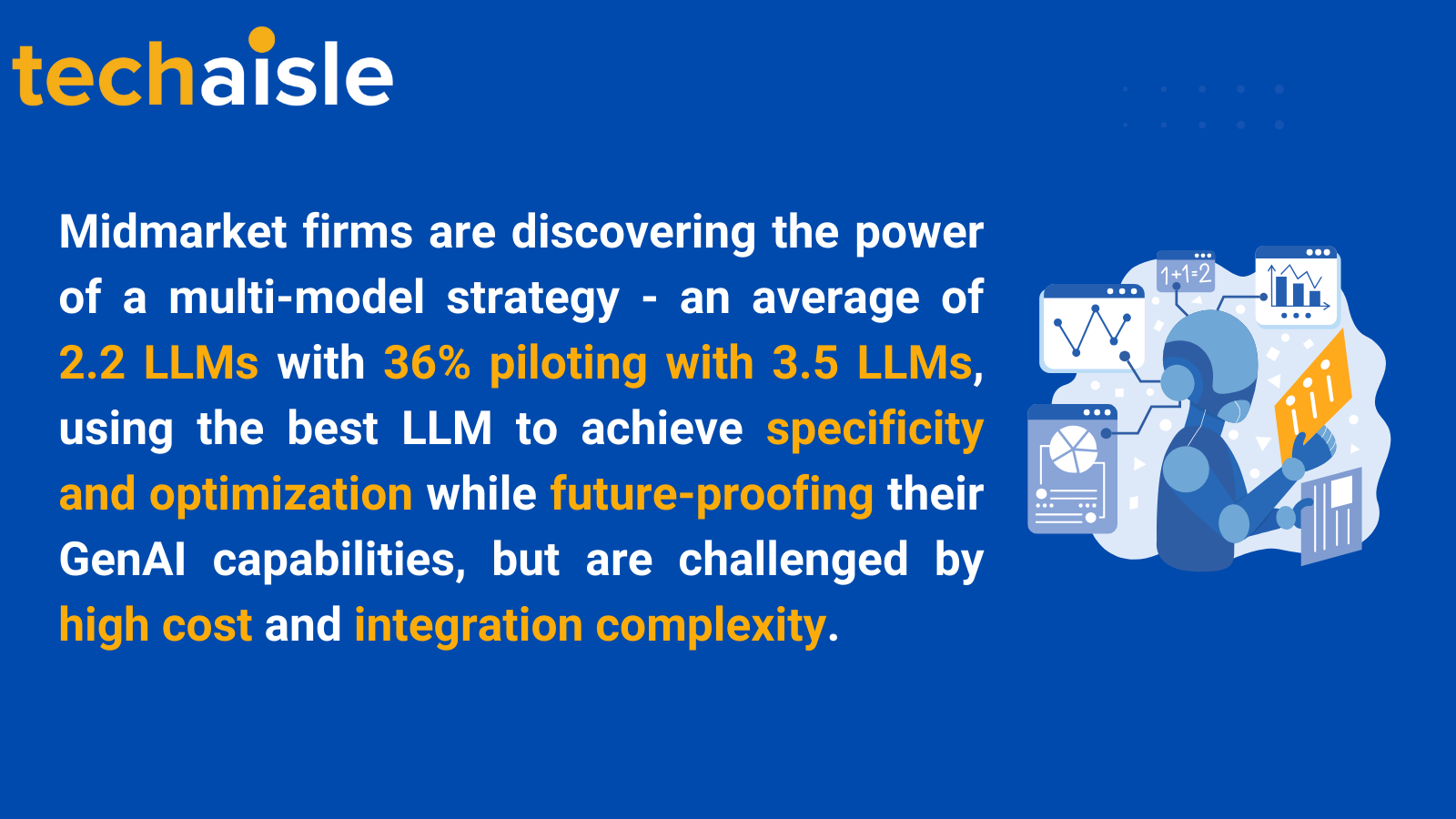
Why Multi-Model Makes Sense for Midmarket Firms
The journey from experimentation to full-fledged adoption requires a strategic approach, and many midmarket firms are discovering the need to experiment with and utilize multiple GenAI models. There are several compelling reasons why midmarket firms believe that a multi-model strategy might be ideal:
Specificity and Optimization: Various LLMs specialize in different tasks. Midmarket firms believe they can benefit from a multi-model strategy, using the best-suited model for each purpose. This may enhance efficiency and precision across a broad spectrum of use cases. Since GenAI can reflect biases from its training data, a multi-model approach also serves as a safeguard. Combining models informed by diverse datasets and viewpoints ensures a more equitable and efficient result.
Future-Proofing: LLMs are rapidly advancing, offering a stream of new features. Without a visible roadmap from LLM providers, midmarket firms hope to benefit from using various models to stay current with these innovations and remain flexible in a dynamic market. As business requirements shift, a diversified model strategy enables modification of their GenAI tactics to align with evolving needs. This strategy permits businesses to expand specific models to meet increasing demands or retire outdated ones as necessary.
Despite the benefits, midmarket firms are also experiencing challenges
High Cost: LLMs have a high price tag, particularly for smaller midmarket companies. Creating and maintaining an environment that supports multiple models leads to a substantial rise in operational expenses. Therefore, a small percentage of midmarket firms are conducting a thorough cost-benefit analysis for every model and optimizing the distribution of resources to ensure financial viability over time. Managing and maintaining multiple LLMs is time-consuming, as different models have varying data formats, APIs, and workflows. Developing a standardized approach to LLM utilization across the organization has been challenging, and a lack of engineering resources has surfaced.
Specialized Skills: Deploying and leveraging multiple LLMs necessitates specialized skills and knowledge. To fully capitalize on the capabilities of a diverse GenAI system, it is essential to have a team skilled in choosing suitable models, customizing their training, and integrating them effectively. Midmarket firms are investing in training for their current employees or onboarding new specialists proficient in LLMs.
Integration Challenges: Adopting a multi-model system has benefits but can complicate the integration process. Midmarket firms are challenged to craft a comprehensive strategy to incorporate various models into their current workflow and data systems. The complexity of administering and merging numerous GenAI models necessitates a solid infrastructure and technical know-how to maintain consistent interaction and data exchange among the models.
Midmarket Firms Intend to Adopt DataOps to Develop GenAI Solutions Economically
While large enterprises have shown how effective DevOps can be for traditional app development and deployment, midmarket firms notice that conventional DevOps approaches may not fit as well for emerging AI-powered use cases or GenAI. Techaisle data shows that only half of the midmarket firms currently have the necessary talent in AI/ML, DevOps, hybrid cloud, and app modernization. Although DevOps is great for improving the software lifecycle, the distinct set of demands introduced by GenAI, primarily due to its dependence on LLMs, poses new hurdles.
A primary focus for midmarket firms is ensuring a steady user experience (UX) despite updates to the foundational model. Unlike conventional software with updates that may add new features or bug fixes, LLMs are built to learn and enhance their main functions over time. As a result, while the user interface may stay unchanged, the LLM that drives the application is regularly advancing. However, changing and or even swapping out these models can be expensive.
DataOps and AnalyticsOps have emerged as essential methodologies tailored to enhance the creation and deployment of data-centric applications, much like those powered by GenAI. DataOps emphasizes efficient data management throughout development, ensuring the data is clean, precise, and current to train LLMs effectively. Conversely, AnalyticsOps concentrates on the ongoing evaluation and optimization of the GenAI applications' real-world performance. Through persistent oversight surrounding user interaction, DataOps and AnalyticsOps empower midmarket firms to pinpoint potential enhancements within the LLM model without requiring extensive revisions, facilitating an incremental and economical methodology for GenAI enrichment. Ultimately, midmarket firms are considering adopting DataOps and AnalyticsOps with a strategic intent to adeptly handle the intricacies inherent in developing GenAI solutions. By prioritizing data integrity, continuous performance assessment, and progressive refinement, these firms hope to harness GenAI's capabilities cost-effectively.
Final Techaisle Take
The success of GenAI implementation probably hinges on a multi-model strategy. Firms that effectively choose, merge, and handle various models stand to fully exploit GenAI's capabilities, gaining a considerable edge over competitors. As GenAI progresses, strategies to tap into its capabilities must also advance. The key to future GenAI advancement is employing various models and orchestrating them to foster innovation and success.